We’re living amidst an explosion of risk related to fraud, money laundering, terrorist financing, and data privacy.
Since 2018, the cost of global cybercrime is estimated to have reached over $1 trillion, according to a study by McAfee and the Centre for Strategic and International Studies (CSIS), which is an increase of more than 50% in two years. An INTERPOL report projects that this trend is getting significantly worse as cybercriminals exploit the fear and uncertainty caused by the unstable social and economic situation created by COVID-19.
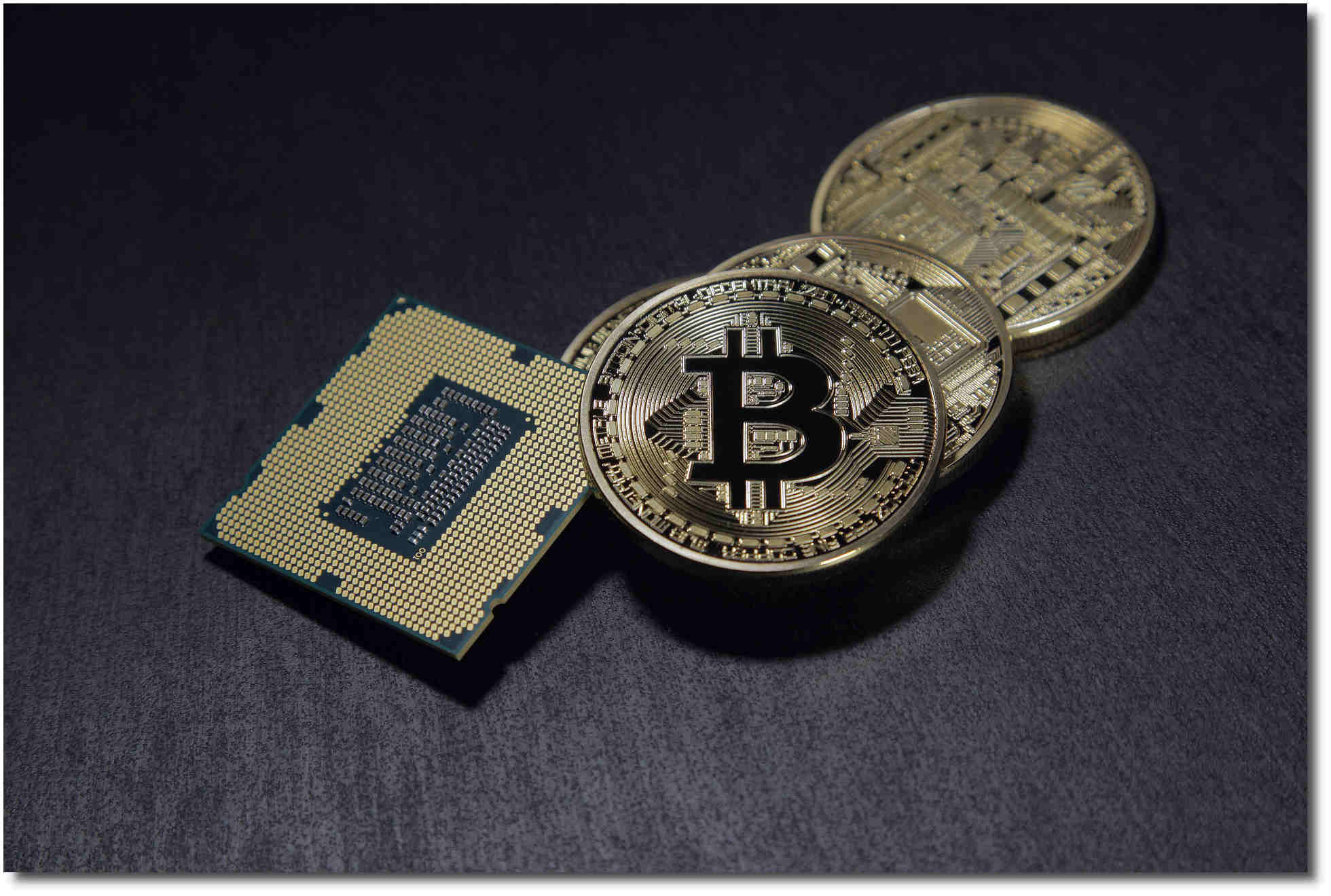
- Global cybercrime costs reached $1 trillion since 2018, i.e., an increase of 50% in two years
- 92% of target organisations reported negative effects
- 56% of organisations do not have a plan to prevent cyber incidents, and of those that do, only 32% believe it is effective
Innovation and Benefits for the Customer
Inspirient's solution for fraud detection enables our customers to detect fraudulent behavior before any financial harm is done. The bank is notified about suspicious processes within minutes and can then prevent unauthorized transactions. Inspirient learns during the interaction with the bank’s fraud experts, so the amount of false positives detected is gradually decreasing. This protects customers from financial and reputational damages and lowers operative risk in the long run.
For one of the Top 10 international banks, Inspirient's Automated Analytics Engine helped them identify a network of cybercriminals before any incident had occurred by detecting anomalous account behaviour.
In detail:- Inspirient's engine automatically scans high volumes of financial data for suspicious account behavior and notifies the risk team in time to act
- By connecting multiple data sources, including monetary transations and account logs, Inspirient's engine was able to detect anomalous correlations
- Through a hybrid approach of rules, unsupervised machine learning, and anomaly detection, the engine is able to detect previously unknown fraud behaviour
- The engine employs a feedback loop to learn from the risk team to lower the rate of false alarms over time
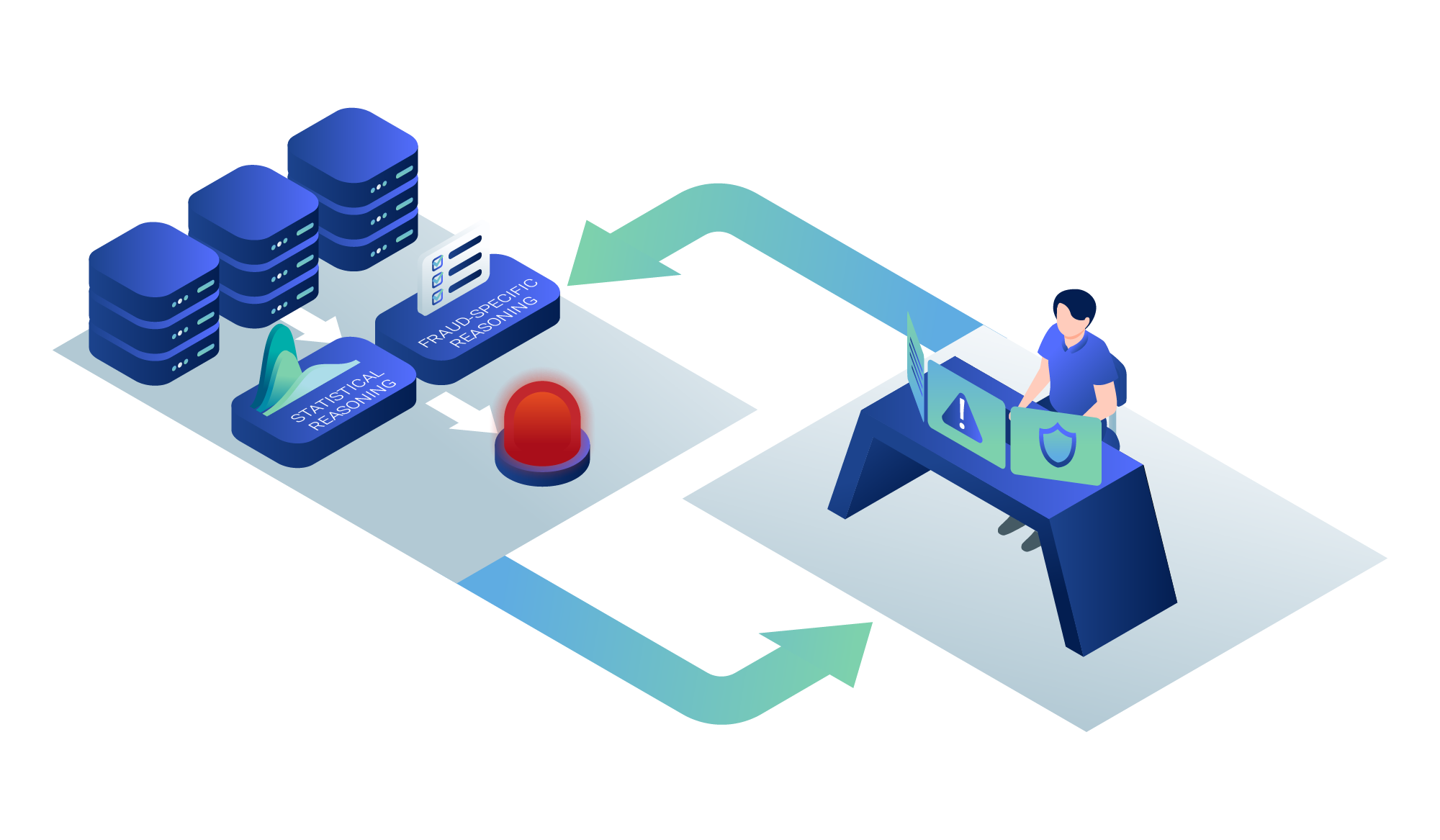
Usage of Automated Analytics
The Inspirient Automated Analytics Engine automatically scans the financial data through its high-speed comprehensive anomaly scan. Within minutes, the system is able to detect known and unknown fraudulent patterns, also custom patterns can be added. To this end, suspicious account behavior is immediately identified and the risk unit of the bank notified in time so unauthorized transactions can be prevented before they occur. Further, the expert knowledge of analysts is fed back into the system through a feedback loop, combining human instinct and experience with machine speed. This enables a constant improvement of the system, as it gets more and more accurate everyday through the feedback it receives.

- Financial data automatically scanned within minutes to detect rule-based exceptions and statistical anomalies
- Suspicious account behavior and transactions detected and classified by priority
- Functional team immediately alerted on detection of a critical issue in time to block transaction
Conclusion
The case above describes how Inspirient's Automated Analytics Engine for financial fraud detecion and prevention safeguards digitalization of financial services by protecting financial institutions and their customers from fraud. This lowers insurance cost connected to fraud of banks and strengthens the trust of their clients. Also, it allows banks to fully leverage digitization and deliver value to their customers through strengthened protection mechanisms.
Further reading
- Frank on Fraud. Top 10 Fraud Types for 2017 Based on Losses.
- PYMNTS.com. Why Fraudulent Account Takeovers Are Adding Up.
The Inspirient Automated Analytics Engine automates the entire data analytics process end-to-end: From the assignment of input data, pattern and outlier detection, automated visualization of patterns, weak points and opportunities to automatic generation of textual explanations and recognition of the underlying relationships and rules. Most other analytics solutions rarely include these textual explanations and observations regarding the underlying data relations, which are both critical to provide a deeper level of analysis and more actionable conclusions.