Driving Digitalization With AI-enabled Analytics
- The German railway company Deutsche Bahn digitally collects maintenance data using an app
- Data was collected from over 5,000 stations operated by DB Station&Service AG
- Our agile insight discovery and prioritization workshop surfaced valuable insights, e.g., drivers of costs and potential for savings
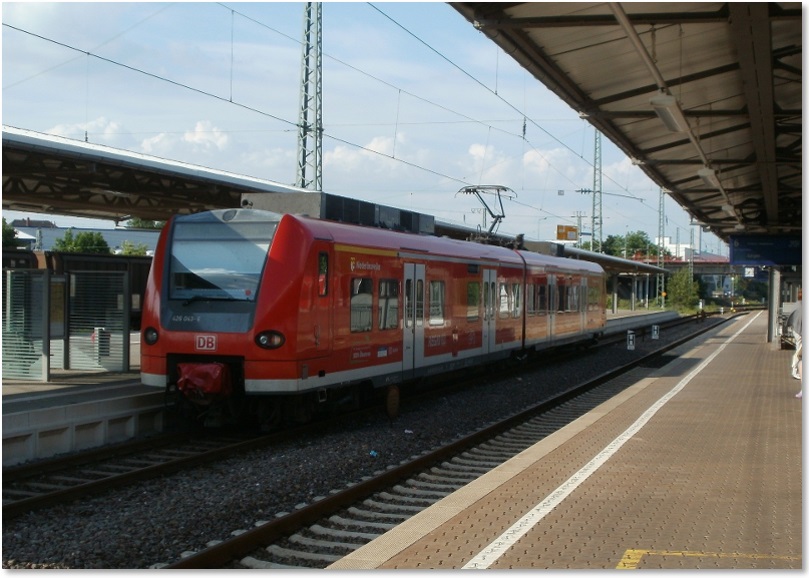
- ~5,400 train stations
- ~6,500 monthly maintenance checks
- ~260,000 total elements to inspect
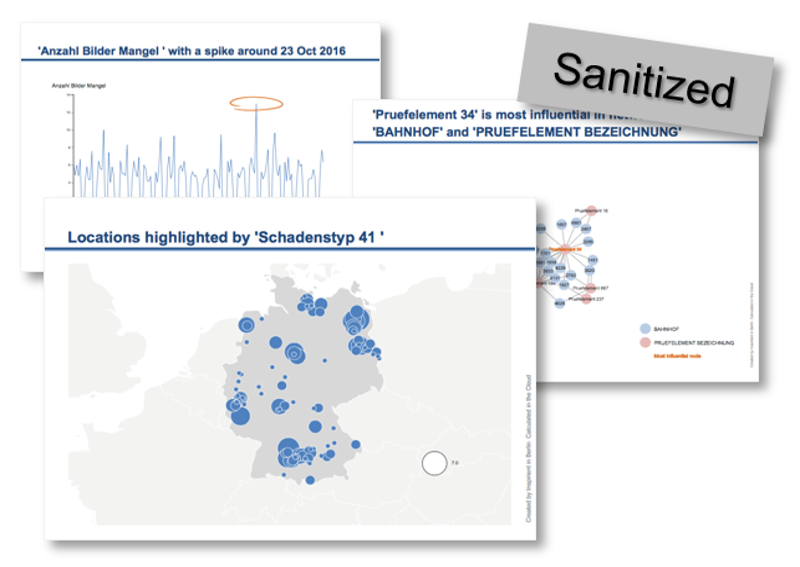
- Identified geographical hotspots for specific damage types
- Discovered station-specific maintenance checks
- Detected spikes over time in number of documented defects
Innovation and Benefits for the Customer
Through an agile, AI-enabled workshop, Deutsche Bahn were able to review patterns and abnormalities relevant to their business that were previously inaccessible from their data with their business intelligence tools. Additionally, a shift in the customer's analytics process took place — for the first time they could take a data-driven business-first approach. This allowed decision makers from Deutsche Bahn to guide and intervene in the analytics process much earlier on, at a point in the process where their intuition and business experience was most valuable.
Enablement through AI-enabled Analytics Workshops
The Inspirient Automated Analytics Engine was used to automatically process, join, and analyze two sets of raw data that were available in CSV format. The two datasets combined consisted of more than 1 million data points. Inspirient automatically scanned the data for relevant patterns and visualized them on management-ready slides, which could be discussed directly in the prioritization workshop with Deutsche Bahn management, without any human work necessary in preparing for this meeting. The slides were then jointly reviewed and discussed by decision makers and analysts from Deutsche Bahn. Around one third of the findings presented by Inspirient were surprising and previously unknown to the company. Among these findings were unknown regional hotspots for certain types of property damage, suggesting that the exchange of materials in specific regions would reduce damage costs. Furthermore, certain machinery prone to malfunctions could be identified, as well as certain peculiarities of maintenance processes in the field, thus isolating drivers of maintenance costs.
Conclusion
This use case is an example of how Inspirient's automated analyses support digitalization and human decision making in large enterprises and leads to increased analytical agility. Inspirient quickly revealed previously unknown and value-adding insights. This enabled decision makers to react quickly, making full use of their expert knowledge while taking data-driven decisions for their business.
Further reading
- Bitkom (2017). Entscheidungsunterstützung mit Künstlicher Intelligenz. Page 175.